Comparison and clustering of several spectral densities
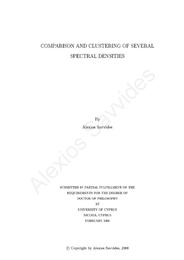
Ημερομηνία
2008-05Συγγραφέας
Savvides, AlexiosΕκδότης
Πανεπιστήμιο Κύπρου, Σχολή Θετικών και Εφαρμοσμένων Επιστημών / University of Cyprus, Faculty of Pure and Applied SciencesPlace of publication
ΚύπροςCyprus
Google Scholar check
Keyword(s):
Metadata
Εμφάνιση πλήρους εγγραφήςΕπιτομή
Θεωρούμε το πρόβλημα εκτίμησης και σύγκρισης πολλών φασματικών συναρτήσεων πυκνοτήτων πιθανότητας, εστω G. Υποθέτουμε ότι οι G-1 από αυτές συνδέονται με την τελευταία μέσω ενός κατάλληλου ημιπαραμετρικού εκθετικού μοντέλου. Από τις ασυμπτωτικές ιδιότητες του περιοδογράμματος, αναπτύσσουμε παραμετρική συμπερασματολογία με βάση την σχετική πιθανοφάνεια για ένα ημιπαραμετρικό μοντέλο. Τα αποτελέσματα μπορούν να εφαρμοστούν σε μια πληθώρα καταστάσεων συμπριλαμβανομένου των γραμμικών διαδικασιών. Προσομοιώσεις και ανάλυση δεδομένων υποστηρίζουν περαιτέρω τα θεωρητικά αποτελέσματα. Η νέα μεθοδολογία εφαρμόζεται σε χρονοσειρές για ανάλυση κατά συστάδες. Γενικές μεθόδοι για την ανάλυση κατά συστάδες στηρίζονται στον υπολογισμό μιας απόστασης μεταξύ δύο ή περισσότερων χρονοσειρών. Νέες αποστάσεις προτείνονται και ο υπολογισμός τους βασίζεται στους συντελεστές Fourier της λογαριθμικής φασματικής συνάρτησης πυκνότητας πιθανότητας. Οι συντελεστές εκτιμώνται με βάση το προαναφερθέν ημιπαραμετρικό μοντέλο. Οι καινούργιες μετρικές προσδιορίζουν το διαχωρισμό των δεδομένων καλύτερα σε σχέση με τις ήδη υπάρχουσες. Consider the problem of estimating and comparing several spectral densities, say G, and assume that the first G-1 of them are related with the last one by an exponential model. Based on the asymptotic properties of periodogram ordinates, we develop parametric likelihood inference for this model. More specifically, we study in detail the asymptotic behaviour of the maximum likelihood estimator under the semiparametric model. The results can be applied in a variety of situations including linear processes. Simulations and data analysis support further the theoretical findings. The new methodology is applied to time series clustering. Methods for clustering are based on the calculation of suitable similarity measures which identifies the distance between two or more time series. New measures of distance are proposed and they are based on the so--called cepstral coefficients which carry information about the log spectrum of a stationary time series. These coefficients are estimated by means of the semiparametric model which was discussed earlier. After estimation, the estimated cepstral distance measures are given as an input to a clustering method to produce the disjoint groups of data. Simulated examples show that the method yields good results, even when the processes are not necessarily linear.