Testing serial dependence by the distance covariance function
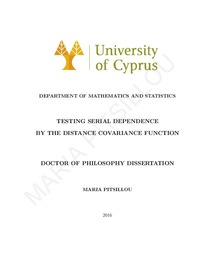
Date
2016-11Author
Pitsillou, Maria N.Publisher
Πανεπιστήμιο Κύπρου, Σχολή Θετικών και Εφαρμοσμένων Επιστημών / University of Cyprus, Faculty of Pure and Applied SciencesPlace of publication
ΚύπροςCyprus
Google Scholar check
Keyword(s):
Metadata
Show full item recordAbstract
Τα τελευταία χρόνια έχει μελετηθεί εκτενώς η εξάρτηση τυχαίων μεταβλητών υιοθετώντας την ένοια της συνάρητης distance covariance (συνδιακύμανση αποστάσεων). Πρόκειται για ένα νέο μέγεθος εξάρτησης, το οποίο έχει εισαχθεί από τους Székely et al. (2007). Πρόσφατα ο Zhou (2012) έχει επεκτείνει την έννοια του distance covariance στις χρονοσειρές. Ωστόσο, έκτοτε ένας περιορισμένος αριθμός άρθρων έχει μελετήσει τις ιδιότητες του νέο αυτού μεγέθους εξάρτησης. Στην παρούσα διατριβή αναπτύσσουμε μία μεθοδολογία για έλεγχο ανεξαρτησίας στις χρονοσειρές βασισμένη στη συνάρτηση distance covariance. Η σημαντικότητα ενός τέτοιου ερευνητικού θέματος προκύπτει από το γεγονός ότι η συνάρτηση distance covariance – και η κανονικοποιημένη της μορφή, η λεγόμενη distance correlation – μπορούν να προσδιορίσουν ενδιαφέρουσες σχέσεις μεταξύ των δεδομένων, τις οποίες η κλασσική συνάρτηση αυτοσυσχέτισης δεν ανιχνεύει, εκτός και αν τα δεδομένα ακολουθούν την κανονική κατανομή ή/και είναι γραμμικώς συσχετισμένα. Εξετάζοντας αρχικά την μονοδιάστατη περίπτωση, κατασκευάζουμε έναν έλεγχο ανεξαρτησίας με στατιστικό τύπου Box-Pierce . Σε αντίθεση με τα κριτήρια Box-Pierce και Ljung-Box, ο αριθμός των χρονικών υστερήσεων που χρησιμοποιείται στην κατασκευή του προτεινόμενου στατιστικού δεν είναι σταθερός αλλά αυξάνεται με το δειγματικό μέγεθος της χρονοσειράς. Επιπλεόν, επεκτείνουμε την μεθοδολογία αυτή σε πολυδιάστατες χρονοσειρές ορίζοντας αρχικά την την συνάρτηση distance covariance υπό μορφή πίνακα. Ο πίνακας distance covariance παρέχει πληροφορίες σχετικά με τις σχέσεις που δυνατόν να υπάρχουν μεταξύ των διάφορων συνιστωσών της χρονοσειράς. Με βάση αυτό το νέο μέγεθος εξάρτησης κατασκευάζουμε έλεγχο ανεξαρτησίας για πολυδιάστατες χρονοσειρές. Το στατιστικό που προκύπτει έχει την μορφή του πολυδιάστατου στατιστικού Ljung-Box, βασισμένο στους πίνακες distance covariance παρά στους κλασσικούς πίνακες αυτοσυσχέτησης. Στο τελευταίο μέρος της εργασίας αυτής παρουσιάζουμε το R πακέτο dCovTS. Μέχρι τώρα, δεν υπάρχει κάποιο αντίστοιχο πακέτο στην R για υπολογισμό της συνάρτησης distance covariance στις χρονοσειρές. Έτσι, το προτεινόμενο πακέτο παρέχει συναρτήσεις που υπολογίζουν και σχεδιάζουν τις συναρτήσεις distance covariance και distance correlation τόσο για μονοδιάστατες όσο και για πολυδιάστατες χρονοσειρές. Επιπρόσθετα, περιλαμβάνει συναρτήσεις που αφορούν στους ελέγχους ανεξαρτησίας όπως εξηγήθηκαν πιο πάνω και παρουσιάζονται σε αυτή την εργασία. Η παρουσίαση του πακέτου και των λειτουργίων του γίνεται μέσω διάφορων πραγματικών δεδομένων. There has been a considerable recent interest in measuring dependence by employing the concept of distance covariance function, a new and appealing measure of dependence for random variables, introduced by Székely et al. (2007). This tool has been recently extended to time series analysis by Zhou (2012), but since then a limited number of works are discussing its properties. In this thesis, we develop a testing methodology based on distance covariance in the context of dependent data, and especially in time series. This is an important research topic because distance covariance - and its normalized form, the so-called distance correlation - can identify interesting links among the data, whereas the traditional correlation coefficient cannot unless the data are Gaussian and/or linearly related. Considering the univariate case, we construct a Box-Pierce type test statistic based on distance covariance for examining independence. Compared to the usual Box-Pierce test statistic - and its modified version, the Ljung-Box test statistic - the number of lags used for the construction of the proposed test statistic is not constant but grows with the sample size. Moreover, we extend the notion of distance covariance to multivariate time series by defining its matrix version. The information contained in this matrix is useful for identifying any possible relationships within and between the time series components. Based on this new concept, we introduce a multivariate Ljung-Box type test statistic with an increasing number of lags, suitable for testing independence. The contributed R package dCovTS is also introduced. There is no available package in the literature regarding the distance covariance theory in time series. The proposed package provides functions that compute and plot distance covariance and correlation functions for both univariate and multivariate time series. Additionally, it includes functions for testing serial independence based on the proposed methodology presented in this thesis. In the last part of the thesis, we discuss in detail the implementation of the package with several real data examples.